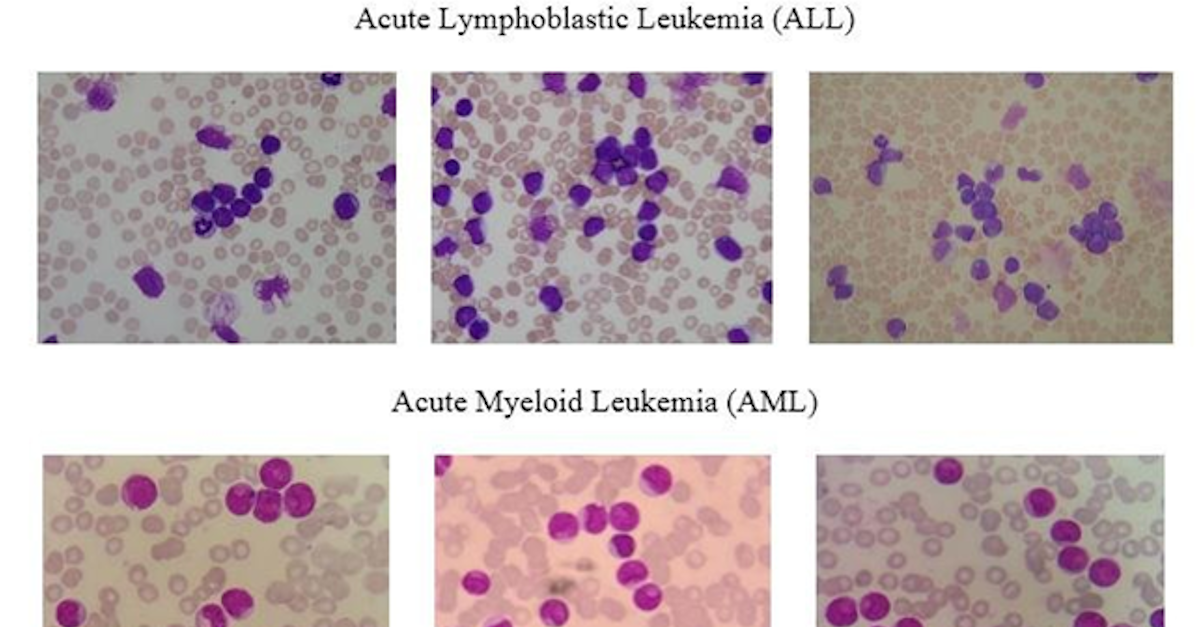
Acute Myeloid Leukemia: A general introduction
In this article, I will describe briefly what AML is, the current classification, current therapies available, and open questions. In the next articles, I will discuss diagnosis, risk classification with a particular eye about machine learning models and approach to them. Some of the presented approaches have been developed for other leukemia subtypes, but the principles are the same and can be applied to AML. In the next articles, it will be a focus also on limitations and perspectives in leukemia.
Acute myeloid leukemia (AML) is a serious disease (where more than 20 % of the blood cells are cancerous cells or myeloblasts), and if left untreated is becoming rapidly fatal. Diagnosis is often obtained by noticing abnormalities in the blood count during a blood test. The diagnosis is completed by microscopical analysis of blood smear and often bone marrow aspiration (microscopical or flow cytometry analysis). AML arises from a disorder of hematopoietic stem and progenitor cells (HPSCs), this is generally caused by genetic abnormalities (inherited or acquired). Despite the fact that it can occur at any age; an AML diagnosis at an elderly age is considered more likely. It is considered one of the deadliest cancers (5-year overall survival inferior to 30%) and with a high relapse rate and high rate of resistance to the therapy. AML is leading to primary infiltration of the hematopoietic organs: bone marrow, spleen, and lymph nodes. This is resulting life-threatening complications such as immunodeficiency, thrombocytopenia, and anemia (1). Despite the emerging of new technologies and insight into AML biology the standard therapy has not changed much in the last decades.
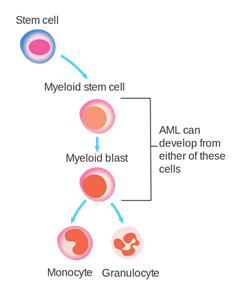
Figure 1. HPSCs stage where AML can develop. Source: (2).
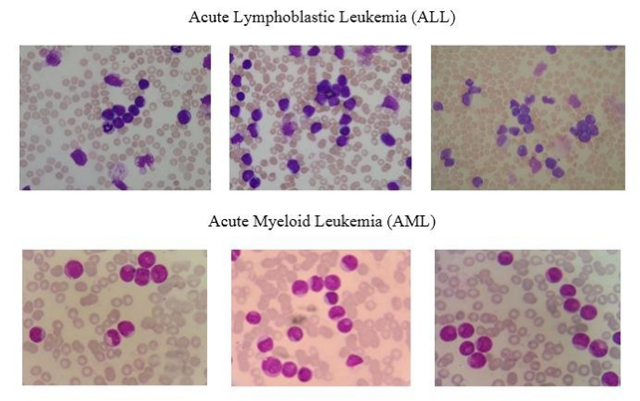
Figure 2: example of blood smear. Figure source: (3).
Traditionally, AML has been classified according to morphology and the immunophenotype of the myeloblasts. Since the advent of omics technologies, it has been questioned the idea that AML is a homogeneous disease: the current view considers AML as a heterogeneous group of neoplastic disorders. The new classification is taking into account the genetic anomalies, for instance, chromosome translocation, fusion genes, and other abnormalities. These genetic abnormalities have a great impact on the prognosis and have to be taken into account in the choice of the treatment protocol. World Health Organization (WHO) has nowadays classified AML in 6 different categories or subtypes (4):
1. AML with recurrent genetic abnormalities. It accounts for around 20–30 % of patients diagnosed with AML and presents a particular profile of chromosomal translocation or inversions.
2. AML with myelodysplasia-related changes. Generally patient with a myelodysplastic syndrome which evolves in AML
3. Therapy-related myeloid neoplasm. AML disease evolves as an effect of chemotherapy or radiotherapy.
4. Acute myeloid leukemia, not otherwise specified. AML cases that are not classified in the other categories
5. Myeloid sarcoma. A cancer mass of myeloblasts is localized in a different site from the bone marrow
6. Myeloid proliferations associated with Down syndrome. Individuals who have Down syndrome are known to have an increased risk of AML.
There are also other classification systems that are based on prognosis and risk stratification but they are also taking into account genomic alterations.
The AML therapy is composed of two phases: induction (with the scope of complete remission or reducing the myeloblast cell to an undetectable level) and consolidation (to eliminate the undetectable residual of leukemia to prevent an early relapse). Conceptually, consolidation therapy is aimed to eradicate the residual myeloblast and cancer stem cells. Young AML patients are treated with intensive chemotherapy or according to the prognosis with bone marrow transplantation (normally transplantation is used in the case of induction therapy is ineffective). However, more than 50 % of AML newly diagnosed patients are older than 65 years, which are not eligible for aggressive chemotherapy and present a poor prognosis (5-year survival probability less than 10 %). Indeed, there is the need to develop new therapies for a large part of the AML of patients (5). Latest studies (conducting next-generation sequencing) showed that a shortlist of gene mutations occurs in the majority of the AML patients (for instance: FLT3, NPM1, DNMT3, IDH1, and IDH2) (6,7). Moreover, other studies have also noticed mutation in WT1 and TP53 which are tumor suppressor genes. These mutations can be also predictive for the outcome: for example, patients with NPM1 mutation are more responsible for chemotherapy while patients with NPM1 and FLT3 mutation have a less favorable prognosis with increased risk of relapse (8).
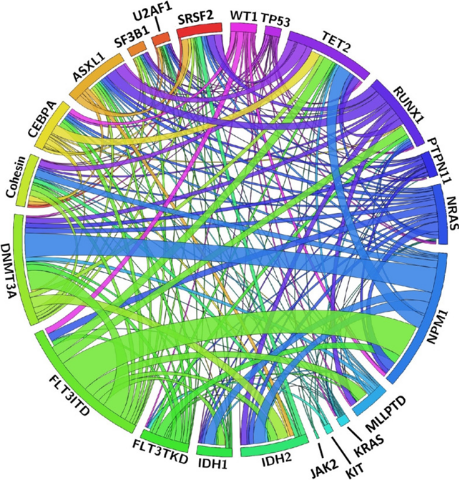
Figure 3. the circular plot is showing the relative frequency and pairwise co-occurrence of genetic alterations in a large cohort. Figure source: (9).
New therapies have been developed based on these mutations which have been approved by the FDA in the last years (for example, a drug against FLT3 or IDH mutated AML). Since FLT3 mutation leads to a more aggressive phenotype and lower survival, different research groups have been interested in the research of effective drugs against FLT3. AML patients have benefited from the first and second generation of FLT3 inhibitors with improved survival. However, the patients which present these mutations and meet the criteria for these new treatments are about 50%, leaving the need of new treatments for the excluded patients. Moreover, since many of these patients are elderly, we need drugs that are less toxic (more tolerable) and could be combined with other drugs (conventional or not)
Among the new therapies in development, there are drugs that are acting on specific signal pathways. As an example, there are drugs targeting apoptosis through BCL-2 inhibition. A BCL-2 is a gene that promotes cell survival regulating the apoptotic pathway and has shown to play an important role in myeloblast resistance to chemotherapy. For these reasons, there is in a study a selective inhibitor of BCL-2. Another treatment in evaluation is against MDM2 which is another gene implicated in the escape from apoptosis. Apart from apoptosis, there are studies evaluating drugs directed to proliferation, differentiation, and other pathways. As other examples, there are drugs in development against the hedgehog pathway, CDK9, and so on (10).
Another approach is based on the epigenetic regulation of DNA. Gene expression in the nucleus is regulated by chemical modification of the DNA such as methylation or acetylation of the histone (proteins bound to the DNA). The latest researches have shown that these processes are unbalanced in the myeloblasts. DNMT3, IDH1, and IDH2 mutations are leading to methylation unbalance in AML. FDA has approved Azacitidine or Decitabine that has the first class of epigenetic drug, these drugs showed to inhibit the methylation of DNA. However other drug targeting epigenetic processes are in the evaluation as BET inhibitors, LSD1 inhibitors, and DOT1L inhibitors (10).
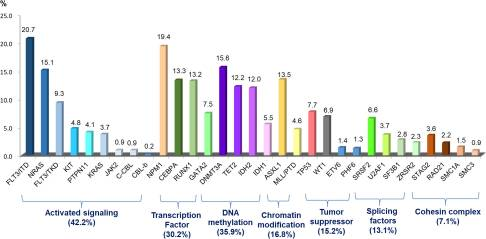
Figure 4: molecular alteration and signaling pathway. Figure source: (9).
In the last years, monoclonal antibodies (MoAb) have been widely used in the treatment of different cancers. Since myeloblasts are present in the blood and the bone marrow they are a suitable target for MoAb. MoAb generally functions by binding on cell surface antigen and then signaling the cell to the immune system kills the malignant cell. The use of MoAb is limited by two principal issues, the first most of the suitable antigens are also expressed by healthy immune cells. Second, MoAbs have shown to be ineffective in AML if they are not conjugated with toxic agents. MoAb anti CD33 has been developed (which is expressed in the 90% of leukemia cells). One of the strategies is to link the anti-CD33 MoAb with a cytotoxic agent (for example calicheamicin, a molecule that is determining damage to the DNA). The principle, in this case, is that the MoAb is bounded to the surface of the myeloblast and once it is internalized the cytotoxic agent kills the cell. Another target for MoAb is CD123, a gene expressed in the vast majority of AML patients. There is also a new approach in MoAb therapies, such as the MoAb designed to cross-link myeloblast to immune cells (typically T-cells or NK-cells). This new approach is showing promising results and is in clinical evaluation (10).
A different approach in MoAb is directed to reactivate the immune system against the myeloblast instead of directly killing the myeloblast. The immune system is conducting immune surveillance against mutated cells. Malignancies occur when the cancer cells are able to escape the immune system. Often this happens suppressing the immune response against cancer. In the last years, a growing interest is directed on what are called immune checkpoints. Immune checkpoints are proteins that are able to regulate the immune response, negative immune checkpoints are dampening the immune response while positive checkpoints are promoting immune response. In different types of malignancies, MoAb against negative immune checkpoints is entering clinics showing very promising results. As examples, there are MoAb directed against CTLA4, PD-1, CD47 (10).
Chimeric Antigen Receptor (CAR) T-Cell Therapy is a different approach exploiting the immune system against the tumor. CAR T-cells are genetically engineered cells to express particular molecules on the surface to exert tumor-lytic activity. In many different cancers, the CAR T-Cell Therapy has shown appreciable results, leading to the idea of developing a similar approach to AML (10).
The study of the biology of AML is leading to a better comprehension of the disease. This increasing knowledge has led researchers to identify new targets in AML and it has been followed by the development of new molecules. Indeed, there are a lot of ongoing clinical trials that are evaluating the efficacy of these molecules and approaches in AML. A challenge for clinicians is to choose the optimum treatment for the patient, with particular attention to elderly or unfit patients. Clinicians should choose the optimal treatment according to age, comorbidities, and genetic mutations. This requires genome analysis after the diagnosis to choose the optimal treatment. However, after the treatment AML can relapse and present new mutations, then an accurate analysis is necessary to choose the optimal treatment. Deep sequencing analysis has shown that myeloblasts are heterogeneous and a percentage of the primary tumor presents different alterations. Moreover, this subclone can expand and acquire other mutations. These new molecules that can be used to treat relapse cases will lead to newly acquired resistance (as new gene mutation, different alteration in the signaling pathways, and so on) requiring to study of alternative strategies. For example, AML patients treated with FLT3 or IDH2 inhibitors have already developed resistance (different studies showed the different mechanisms of acquired resistance to FLT3 inhibitors, leading to the need for ulterior strategies) (11).
Gene mutations and genomic alteration in AML are the basis of the pathogenesis and the basis for the relapse. Deep sequencing of AML has permitted us to better classify AML in different subgroups. Moreover, the mutation profiles allowed to stratify patients into the risk group and to better predict prognosis. They have also permitted the development of new therapies. These more specific therapies proved to be more tolerable in older patients which cannot be treated with standard chemotherapy. However, this is not enough, the genetic alterations present in AML are beyond the markers we highlighted before. Moreover, some of these mutations arise at different stages of disease (primary, relapse, after treatment), meaning that our stratification based on these genetic abnormalities is still inaccurate. The discovery of new mutations or other alterations (like in signaling pathways) in primary tumors or in relapses would allow the development of new primary therapies or for the secondary lines. Another important aspect of these gene mutations is the possibility of early discovery relapse through molecular analysis. There is also interest in the epigenetic landscape which could lead to other promising therapy and can be used as an early marker for relapse. At the current state, we have less knowledge on the epigenetic landscape in AML during progression and its role in relapse and resistance to therapy. At the same time immunotherapy is still in development, notwithstanding it is showing promising results there are still some black spots we have to address. New therapies or a combination of them can lead to malignancy eradication during the preleukemic stage or before relapse. Another challenge that has to be addressed is marker identification of leukemia stem cells which are remaining after therapy and prompting the relapse. The gain of knowledge in these fields is the basis for personalized medicine. It is expected that the right combination of these therapies will be chosen according to the specific subgroup of patients. Thus, there is a need for new preclinical study to identify the right subgroup for the right combination of therapies. At the same time, it is also necessary to improve the trial clinical design (12).
An open question is how myeloblasts remodel the bone marrow to create a permissive niche to sustain tumor growth. In the same way, AML is leading to metastasis where malignant cells interact with different types of cells. These processes involve many different molecules involved in adhesion, proliferation, and differentiation. To address these last questions, we need to understand interactions with other cells that are present in the bone marrow.
In conclusion, there are still many open questions that have to be addressed by researchers and as discussed in the next articles there is room for machine learning.
following article on medical image analysis with machine learning: here.
following article on machine learning using other data source: here
Selected bibliography:
1. Pievani A, Biondi M, Tomasoni C, Biondi A, Serafini M. Location First: Targeting Acute Myeloid Leukemia Within Its Niche. J Clin Med [Internet]. 2020 [cited 2020 Sep 29];9. Available from: https://www.ncbi.nlm.nih.gov/pmc/articles/PMC7290711/
2. Acute myeloid leukemia [Internet]. Wikipedia. 2020 [cited 2020 Oct 8]. Available from: https://en.wikipedia.org/w/index.php?title=Acute_myeloid_leukemia&oldid=976980044
3. Fatonah NS, Tjandrasa H, Fatichah C. Automatic Leukemia Cell Counting using Iterative Distance Transform for Convex Sets. International Journal of Electrical and Computer Engineering (IJECE). 2018;8:1731–40.
4. Hwang SM. Classification of acute myeloid leukemia. Blood Res. 2020;55:S1–4.
5. Appelbaum FR, Gundacker H, Head DR, Slovak ML, Willman CL, Godwin JE, et al. Age and acute myeloid leukemia. Blood. 2006;107:3481–5.
6. Papaemmanuil E, Gerstung M, Bullinger L, Gaidzik VI, Paschka P, Roberts ND, et al. Genomic Classification and Prognosis in Acute Myeloid Leukemia. N Engl J Med. 2016;374:2209–21.
7. Genomic and Epigenomic Landscapes of Adult De Novo Acute Myeloid Leukemia. N Engl J Med. 2013;368:2059–74.
8. Kirtonia A, Pandya G, Sethi G, Pandey AK, Das BC, Garg M. A comprehensive review of genetic alterations and molecular targeted therapies for the implementation of personalized medicine in acute myeloid leukemia. J Mol Med. 2020;98:1069–91.
9. Hou H-A, Tien H-F. Genomic landscape in acute myeloid leukemia and its implications in risk classification and targeted therapies. J Biomed Sci [Internet]. 2020 [cited 2020 Oct 7];27. Available from: https://www.ncbi.nlm.nih.gov/pmc/articles/PMC7372828/
10. Miyamoto K, Minami Y. Cutting Edge Molecular Therapy for Acute Myeloid Leukemia. Int J Mol Sci [Internet]. 2020 [cited 2020 Sep 29];21. Available from: https://www.ncbi.nlm.nih.gov/pmc/articles/PMC7404220/
11. Green SD, Konig H. Treatment of Acute Myeloid Leukemia in the Era of Genomics — Achievements and Persisting Challenges. Front Genet [Internet]. 2020 [cited 2020 Sep 29];11. Available from: https://www.ncbi.nlm.nih.gov/pmc/articles/PMC7267060/
12. Döhner H, Estey E, Grimwade D, Amadori S, Appelbaum FR, Büchner T, et al. Diagnosis and management of AML in adults: 2017 ELN recommendations from an international expert panel. Blood. 2017;129:424–47.